Moving Average Model
Moving average model of order 1
The moving average
(MA) model is a linear combination of $Z$’s. The MA(1) model is given by:
$$ X_t = \mu + Z_t + \theta Z_{t-1} $$
where $\mu$ is the overall mean, $\theta$ is a moving average coefficient, and $Z_t \overset{i.i.d.}{\sim} N(0, \sigma^2)$.
Note that many books use $-\theta$ in the formula, but we’re following R’s convention with the $+\theta$.
Mean and variance
The expectation is
$$ E(X_t) = \mu + E(Z_t) + \theta E(Z_{t-1}) = \mu $$
Setting $\mu = 0$, the variance is
$$ Var(X_t) = Var(Z_t + \theta Z_{t-1}) = Var(Z_t) + \theta^2 Var(Z_{t-1}) = (1 + \theta^2)\sigma^2 $$
Autocovariance and autocorrelation
The autocovariance function of lag 1 is
$$ \begin{aligned} \gamma_X(1) &= Cov(X_t, X_{t-1}) \\ &= Cov(Z_t + \theta Z_{t-1}, X_{t-1}) \\ &= Cov(Z_t, X_{t-1}) + \theta Cov(Z_{t-1}, X_{t-1}) \\ &= \theta Cov(Z_{t-1}, Z_{t-1} + \theta Z_{t-2}) \\ &= \theta \left[ Cov(Z_{t-1}, Z_{t-1}) + \theta Cov(Z_{t-1}, Z_{t-2}) \right] \\ &= \theta \sigma^2 \end{aligned} $$
Thus the autocorrelation of lag 1 is
$$ \rho_X(1) = \frac{\gamma_X(1)}{\gamma_X(0)} = \frac{\theta}{1 + \theta^2} $$
For time lags of 2 and above,
$$ \begin{aligned} \gamma_X(k) &= Cov(X_t, X_{t-k}) \\ &= Cov(Z_t + \theta Z_{t-1}, X_{t-k}) \\ &= Cov(Z_t, X_{t-k}) + \theta Cov(Z_{t-1}, X_{t-k}) \\ &= 0, \quad k = 2, 3, \cdots \end{aligned} $$
which means
$$ \rho_X(k) = 0,\quad k = 2, 3, \cdots $$
A sample ACF with significant autocorrelation only at lag 1 is an indicator of a potential MA(1) model.
Partial autocorrelation
For the MA(1) model, the PACF is
$$ \phi_{00} = 1, \phi_{11} = \frac{\theta}{1 + \theta^2} = \rho(1), \phi_{kk} = \frac{\theta^k(1-\theta^2)}{1 - \theta^{2(k+1)}} \text{ for } k > 1 $$
The PACF od MA(1) tails off after lag 1.
Simulation in R
We can use the arima.sim()
function to simulate the MA model, specifying the model
parameter as list(ma = theta)
. Of course we can also write our own function to generate a series manually:
|
|
Here’s an example of a simulated MA(1) series with $n=200$ timepoints1. We can see the tail off pattern in the ACF and the cut off after lag 1 in the PACF.
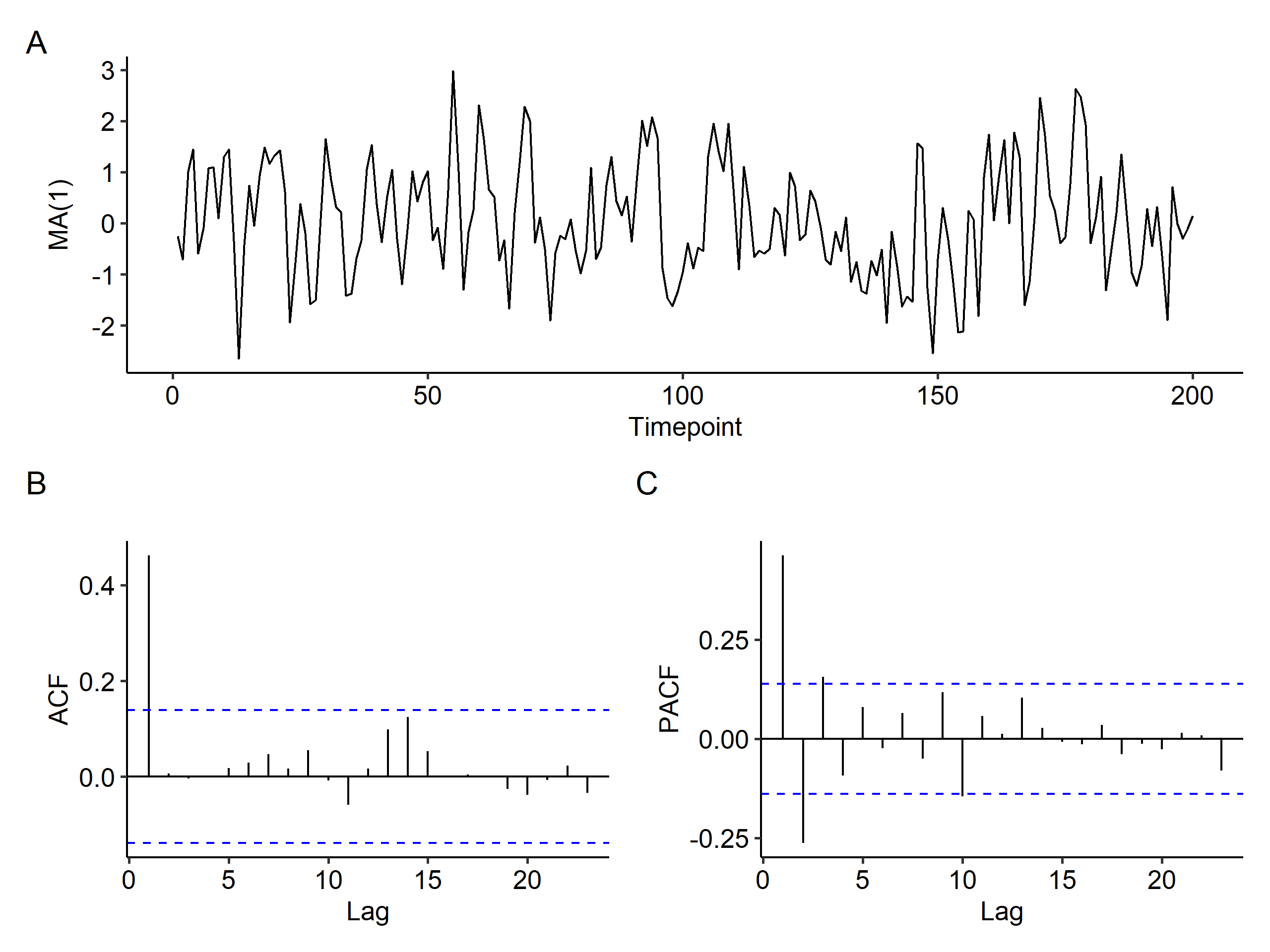
Moving average model of order $q$
The model is
$$ X_t = \mu + Z_t + \theta_1 Z_{t-1} + \theta_2 Z_{t-2} + \cdots + \theta_q Z_{t-q} $$
where the $\theta_j$’s are moving average coefficients and $Z_t$ is $WN(0, \sigma^2)$.
Mean and variance
The terms are all independent, so we don’t have to worry about covariance terms when finding the variance:
$$ E(X_t) = \mu, Var(X_t) = (1 + \theta_1^2 + \theta_2^2 + \cdots + \theta_q^2)\sigma^2 $$
MA(q) is always stationary.
Autocovariance and autocorrelation
The autocovariance for lag 1 is:
$$ \begin{aligned} \gamma_X(1) = &= E\left[(Z_t + \theta_1 Z_{t-1} + \cdots + \theta_q Z_{t-q})(Z_{t-1} + \theta_1 Z_{t-2} + \cdots + \theta_q Z_{t-1-q}) \right] \\ &= (\theta_1 + \theta_2 \theta_1 + \theta_3 \theta_2 + \cdots + \theta_q \theta_{q-1})\sigma^2 \end{aligned} $$
The autocovariance function for lag $k$ is:
$$ \begin{aligned} \gamma_X(1) = &= E\left[(Z_t + \theta_1 Z_{t-1} + \cdots + \theta_q Z_{t-q})(Z_{t-k} + \theta_1 Z_{t-k-1} + \cdots + \theta_q Z_{t-k-q}) \right] \\ &= \begin{cases} (\theta_k + \theta_{k+1} \theta_1 + \theta_{k+2} \theta_2 + \cdots + \theta_q \theta_{q-k})\sigma^2, & k = 1, 2, \cdots, q \\ 0, & k > q \end{cases} \end{aligned} $$
From this we can find the autocorrelation function for lag $k$:
$$ \rho_X(k) = \begin{cases} \frac{\theta_k + \theta_{k+1}\theta_1 + \theta_{k+2}\theta_2 + \cdots + \theta_q\theta_{q-k}}{1 + \theta_1^2 + \theta_2^2 + \cdots + \theta_q^2}, & k = 1, 2, \cdots, q \\ 0, & k > q \end{cases} $$
To summarize the differences between the two models:
Model | ACF | PACF |
---|---|---|
AR(1) | Tail off | Cut off after lag 1 |
AR(p) | Tail off | Cut off after lag $p$ |
MA(1) | Cut off after lag 1 | Tail off |
MA(q) | Cut off after lag $p$ | Tail off |
Invertibility
We’re interested in expressing an MA series as an AR series. AR series are more intuitive because $X_t$ is a linear combination of the past data, and the AR coefficients can be directly interpreted.
Under certain conditions, we can invert an MA series to an AR series. Taking a zero-mean MA(1) series as an example:
$$ X_t = Z_t + \theta Z_{t-1}, \quad Z_0 = 0 $$
$$ \begin{aligned} X_1 &= Z_1 + \theta Z_0 = Z_1 \\ X_2 &= Z_2 + \theta Z_1 = Z_2 + \theta X_1 \Rightarrow Z_2 = X_2 - \theta X_1 \\ X_3 &= Z_3 + \theta Z_2 = Z_3 + \theta(X_2 - \theta X_1) = Z_3 + \theta X_2 - \theta^2 X_1 \\ &\,\vdots \\ X_t &= Z_t + \theta Z_{t-1} = Z_t + \theta X_{t-1} - \theta^2 Z_{t-2} \\ &= \cdots = Z_t + \theta X_{t-1} - \theta^2 X_{t-2} + \cdots - (-\theta)^k X_{t-k} - (-\theta)^{k+1} \underbrace{Z_{t-k-1}}_{\rightarrow Z_0} \end{aligned} $$
By repeated substitutions,
$$ Z_t = X_t + (-\theta) X_{t-1} + (-\theta)^2 X_{t-2} + \cdots + (-\theta)^k X_{t-k} + \cdots \quad \text{if } |\theta| < 1 $$
The above is not always possible. For example, if $\theta > 1$, the current value will be heavily dependent on data faraway in the past, which doesn’t make sense in a time series. Late we’ll explain how to find this condition systematically.
The current $Z_t$ (shock
) is a linear combination of the present and past $X_t$ (returns
). Since the remote value $X_{t-j}$ should have very little impact on the current shock, $|\theta| < 1$. Such an MA(1) model is said to be invertible
.
Instead of checking stationarity, we usually check invertibility for MA series. The invertibility assures the uniqueness of the connection between values of $\theta$ and $\rho(1)$ in MA(1) - for any value of $\theta$, the reciprocal $\frac{1}{\theta}$ gives the same value for
$$ \rho(1) = \frac{\theta}{1 + \theta^2} = \frac{\frac{\theta}{\theta^2}}{\frac{1 + \theta^2}{\theta^2}} = \frac{\frac{1}{\theta}}{1 + \frac{1}{\theta^2}} $$
Generally, an MA model is invertible if it’s equivalent to a converging infinite order AR model, where converging
means that the AR coefficients decrease to 0 as we move back in time.
R code for simulating the MA(1) series.
↩︎1 2 3 4 5
set.seed(1) x <- arima.sim(model = list(ma = 0.7), n = 200, rand.gen = rnorm) plot_time_series(x, x_type = "MA(1)")
Sep 12 | ARMA Model | 8 min read |
Aug 28 | Autoregressive Series | 9 min read |
Nov 17 | Spectral Analysis | 9 min read |
Nov 02 | Decomposition and Smoothing Methods | 14 min read |
Sep 14 | Model Fitting and Forecasting | 15 min read |